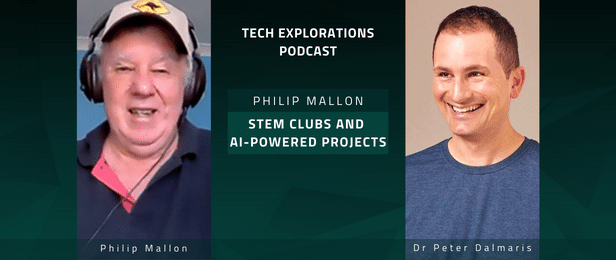
In this episode of Tech Exploration Podcast, we reconnect with Philip Mallon, a retired yet incredibly active industry representative for the Commonwealth Scientific and Industrial Research Organisation (CSIRO) in Australia. Philip shares his journey over the past year, highlighting his involvement in the CSIRO's industry STEM program for schools and his collaboration with local councils such as Blacktown and Parramatta.
Philip begins by explaining the role and significance of the CSIRO, Australia's premier research organization known for groundbreaking innovations like Wi-Fi and the first use of computers in music. He delves into his work with project-based STEM clubs that are designed to extend students' formal STEM education beyond the traditional curriculum. These clubs, operating in schools like Chatswood High School and Ravenswood Girls School, offer students the opportunity to engage in topical projects that connect with real-world issues.
Most discussion revolves around integrating artificial intelligence (AI) in STEM projects. Philip has been working with AI tools like Google's Teachable Machines and Microsoft's Lobe to address practical problems like school waste management. He describes how AI can be used to classify rubbish and provide feedback to students, thereby improving their recycling habits. Philip also touches on edge AI, allowing local data processing on devices like the Raspberry Pi, making the system more reliable even without internet connectivity.
The conversation takes an interesting turn as Philip discusses the use of AI in environmental projects, such as identifying bird species through audio recognition. This project aims to connect students with nature, fostering a deeper appreciation for their environment. He also mentions the use of AI in creative projects, like composing theme songs for local football clubs, which helps students see the practical applications of AI in areas they are passionate about.
Philip emphasizes the importance of making STEM education engaging and accessible. He uses familiar tools like Lego and MakeCode to introduce complex concepts in a way that is relatable to students. He also highlights AI's role as an education assistant, helping students iterate and improve their projects more efficiently.
Towards the end of the episode, Philip reflects on the impact of these STEM clubs on students. He notes that while the clubs are less rigorous than formal education, they provide a valuable, low-stress environment for learning. He shares his approach to gathering feedback from students to ensure they enjoy and benefit from the activities.
Philip concludes with advice for educators: leverage external resources and community partnerships to enhance STEM education. He underscores the importance of teamwork and collaboration, drawing on his experiences working with local councils, libraries, and universities to create a network of support for STEM initiatives.
Join us in this insightful episode as Philip Mellon shares his innovative approaches to STEM education, the integration of AI in practical projects, and the importance of community collaboration in fostering a love for science and technology among students.
1. Introduction
- Timestamp: 00:00 - 00:30
- Peter and Phillip discuss Phillip's busy schedule post-retirement.
2. CSIRO Involvement
- Timestamp: 00:30 - 01:30
- Phillip mentions joining the CSIRO as an industry rep in their industry STEM program for schools.
- CSIRO Explanation
- Timestamp: 01:30 - 02:30
- Phillip explains what CSIRO is: Commonwealth Scientific and Industrial Organization, a premier research organization in Australia.
3. STEM Clubs and Local Councils
- Timestamp: 02:30 - 04:00
- Phillip discusses his work with local councils like Blacktown and Parramatta, running STEM clubs that are project-based and not strictly curriculum-bound.
4. Schools Involved
- Timestamp: 04:00 - 05:00
- Phillip mentions working with Chatswood High School and Ravenswood Girls School, focusing on extending STEM education beyond year 10.
5. Challenges in STEM Education
- Timestamp: 05:00 - 06:00
- Discussion on how older students are often too busy with exams and other activities to participate in STEM clubs during the school term.
6. Reconnection through Blog Post
- Timestamp: 06:00 - 07:00
- Peter and Phillip reconnected through a blog post about ChatGPT and AI.
7. AI in STEM Projects
- Timestamp: 07:00 - 08:00
- Phillip talks about integrating AI into STEM projects, including visual object recognition and using tools like Google Teachable Machines and Microsoft Lobe.
8. Edge AI and Raspberry Pi
- Timestamp: 08:00 - 09:00
- Phillip discusses using Edge AI with Raspberry Pi Pico and ESP32 for making inferences from signals.
9. Bird Call Identification Project
- Timestamp: 09:00 - 11:00
- Phillip describes a project using AI to identify bird calls, aiming to connect students with nature.
10. AI in Music and Football Projects
- Timestamp: 11:00 - 12:00
- Phillip mentions using AI to create theme songs and logos for local football clubs, engaging students by linking AI to their interests.
11. ChatGPT as an Educational Tool
- Timestamp: 12:00 - 14:00
- Discussion on using ChatGPT to help students with coding, explaining code, and improving their projects.
12. Simulation Tools
- Timestamp: 14:00 - 15:00
- Phillip talks about using high-level simulation tools like NetLogo for complex community projects like bushfires and COVID-19.
13. Mind Map Overview
- Timestamp: 15:00 - 16:00
- Peter suggests working through a mind map that Phillip prepared, focusing on the STEM recycling project.
14. Pick Up the Plastic Game
- Timestamp: 16:00 - 17:00
- Phillip describes a student-created game for picking up plastic in the sea, using MakeCode arcade.
15. Rubbish Classifier
- Timestamp: 17:00 - 18:00
- Phillip explains a project using AI to classify rubbish and provide feedback to students on proper recycling.
16. NetLogo for Recycling Economy
- Timestamp: 18:00 - 19:00
- Phillip discusses using NetLogo to simulate the entire recycling economy, showing the life cycle of rubbish.
17. Traffic Light Simulation
- Timestamp: 19:00 - 20:00
- Phillip talks about using NetLogo to simulate and optimize traffic light systems.
18. Student Feedback and Impact
- Timestamp: 20:00 - 21:00
- Phillip mentions getting feedback from students and the positive impact of STEM clubs on their interest and learning.
19. Advice for Educators
- Timestamp: 21:00 - 22:00
- Phillip advises educators to leverage external resources and community support to enhance STEM education.
20. Conclusion and Future Plans
- Timestamp: 22:00 - 23:00
- Peter and Phillip discuss future follow-ups and the importance of networking and collaboration in STEM projects.
Here is a list of resources mentioned by the guest, Philip Mallon, in the transcript:
1. CSIRO (Commonwealth Scientific and Industrial Research Organisation):
- Website: CSIRO
2. Google Teachable Machine:
- Website: Teachable Machine
3. Microsoft Lobe:
- Website: Microsoft Lobe
4. Raspberry Pi Pico:
- Website: Raspberry Pi Pico
5. ESP32:
- Website: ESP32
6. Edge Impulse:
- Website: Edge Impulse
7. ChatGPT:
- Website: ChatGPT
8. Google Bard:
- Website: Google Bard
9. NetLogo:
- Website: NetLogo
10. Scratch:
- Website: Scratch
11. MakeCode Arcade:
- Website: MakeCode Arcade
12. Micro:bit:
- Website: Micro:bit
13. BirdNET:
- Website: BirdNET
14. Lego:
- Website: Lego
15. LittleBits Electronics:
- Website: LittleBits
Tips and Advice from Philip Mallon
1. Leverage External Resources:
- Utilize community resources and industry partners to support STEM education.
- Collaborate with local councils, environmental engineers, and universities to extend the scope of projects.
2. Engage Students with Relevant Topics:
- Connect STEM projects to students' interests, such as creating a theme song for a football club or designing a new logo.
- Use real-world applications and current societal issues to make projects more engaging.
3. Use a Variety of Tools and Technologies:
- Incorporate different tools like AI, simulation models, and coding platforms (e.g., Scratch, MakeCode, Python).
- Utilize AI tools like ChatGPT, Google Teachable Machines, and Microsoft Lobe for various applications.
4. Focus on Project-Based Learning:
- Implement project-based learning rather than strict curricula to foster creativity and practical skills.
- Use hands-on projects like building robots with Lego or creating arcade games to teach complex concepts.
5. Iterative Learning and Feedback:
- Encourage students to iterate on their projects and learn from feedback.
- Reserve time at the end of sessions for reflection and feedback to understand what students have learned.
6. Simplify Complex Concepts:
- Break down complex projects into manageable parts to avoid overwhelming students.
- Use familiar tools and analogies to explain new concepts.
7. Promote Teamwork:
- Foster a collaborative environment where students can work together and learn from each other.
- Encourage students to help each other, especially those who may have more advanced knowledge in certain areas.
8. Incorporate AI in Practical Applications:
- Use AI for practical applications like rubbish classification, bird call identification, and creating music.
- Teach students how to use AI tools to solve real-world problems and improve their environment.
9. Balance Between Fun and Learning:
- Ensure that projects are fun and engaging to maintain student interest.
- Avoid making projects too difficult to prevent frustration and loss of interest.
10. Diagnostic Tools for Troubleshooting:
- Equip students with diagnostic tools to troubleshoot and learn from failed experiments.
- Emphasize that there is no such thing as a failed experiment, only learning opportunities.
11. Network and Share Knowledge:
- Build a network of educators, industry experts, and community members to share knowledge and resources.
- Stay updated with what others are doing in the field to incorporate successful strategies into your own projects.
12. Use AI as an Assistant:
- Teach students to use AI tools like ChatGPT as assistants to help with coding and problem-solving.
- Emphasize the importance of iterating and refining AI-generated solutions.
Peter: Philip Mallon, thank you for joining me in another podcast, another tech exploration podcast. It's been a while since the last time we did one of those. So how have you been?
Philip: Good, thanks. In good health. And also, I've been quite busy. So now I'm retired, I've actually been quite a lot of work.
Peter: I don't think there's such thing for you as retirement. Like you're probably busier now than ever before. What have you been up to in the last year or so?
Philip: In the last year, I've joined the CSIRO as an industry rep in their industry STEM program for schools. So I've been doing that. But I've also been-
Peter: Philip, just sorry to jump in. What is the CSIRO? For those of us who don't know about it.
Philip: The Commonwealth Scientific and Industrial Organization. So in Australia, it's like the premier research, I guess, civilian research organization. It's funded and it's really part of the government, the federal government. And it does fundamental research. But also there's been a lot of spin offs, like Wi-Fi came from the CSIRO.
Peter: Life has never been the same again.
Philip: Yeah, that's right. So even the first use of a computer for making music came from the CSIRO.
Peter: Wow. So the synthesizer?
Philip: Yeah. So things like using computer technology in music is fairly common these days. But it goes back to radio astronomy. And the fact that they were able to identify this as a potential application and demonstrate it, I think is quite interesting.
Peter: Absolutely. Yeah. So now, like it's an amazing organization for anyone that wants to look it up. A lot of good stuff comes out of it. Now you're part of it. Tell us about it.
Philip: Well, with the CSIRO, they're very active in environmental research and in recycling. So what I do in my STEM programs, they're really managing STEM clubs. And it's not only with the CSIRO I'm working with, I'm also working with local councils. And that includes Blacktown Council, and also Parramatta Council. And for each of the organizations, I'm running STEM clubs. So they add on to an existing school program normally. They don't follow a strict curriculum, but they are project-based. And I use bits of my career, experiences from the CSIRO, and things that are happening in society today as a contextual base for the projects that we're working on.
Peter: So the projects you're working on are connected to what's happening in society today. They are, I guess, topical projects. So can you tell us about how those STEM clubs relate to the schools? I think you mentioned that they are connected to a school. Is it like a class from a school where you visit that school and deliver a project program?
Philip: Well, for the two schools that I'm working with, with the CSIRO, one is that Chatswood High School, and the other one's Ravenswood Girls High School or Girls School. Now, both of those schools, the kids are already doing formal STEM education, but they want to take it a bit further. And the other thing about the STEM education, it only tends to go to about year 10. So years upper primary six, then when we go to secondary year seven and eight, it's reasonably active, but then it steers off and goes back to the old methods of working in science and individual subjects. But the kids, they want to take up STEM a bit further than what they get in school. And they can see that perhaps this could get me some more skills, and it might even lead to a career later on.
Peter: Yeah, I've been thinking about that. I guess, at some point in school, kids have to focus on things like exams before entering the university, so they have to focus on curricula, and what's going to be in the exams, right? And those extra STEM curricular activities tend to take second place.
Philip: That's right. So I've noticed, for example, when I have workshops in the school holidays, I get higher attendance. And I also get attendance from the kids who are a bit older, and from the selective schools too. But during the school term, I don't get any of those, I get the younger kids. The older kids are too busy with their homework or with sport or with other activities. So it's quite interesting that there's a different approach, depending on the time that they have available.
Peter: Yeah. It's just the stresses of modern education, I guess the objectives being for many of those kids entry into university and how that system is structured to help them get into a particular university school. We can talk about that. I wanted to also take a step back and just as I was thinking about how did we reconnect because we haven't spoken for a few years. And what happened was that a few weeks ago, I posted something on my blog about chat GPT and artificial intelligence and the latest developments over the last few months. And then you jumped onto the conversation. And that's how we got reconnected. Because you haven't been using chat GPT specifically, but you've been also integrating and including artificial intelligence into your STEM projects. And I remember in a previous conversation, you mentioned something about visual object recognition, for example, which I think is part of your STEM recycling projects. So without getting into specifics yet, we could get into the specifics a bit later. Could you tell us a little bit about the relation between AI and your STEM projects?
Philip: Yeah, I've been working on AI for about three or four years now. But more recently, I've added the newer tools like chat, GDP, and also Google Bob. But the previous work that I was doing was using the tools from Google are called teachable machines. And there's also Microsoft have a tool called libo. And those tools are used in the Chatswood High School, because they said they couldn't manage rubbish. And so I thought artificial intelligence might be one of the potential tools that could be used besides other tools. But the artificial intelligence that I'm looking at, goes back to one not only tools, which is central, like Google's and Microsoft, but also tools that are on the edge. So they're called edge AI. And little tiny computers, like the Raspberry Pi Pico, I'm using those to teach them how to make inferences about some of the signals that I get. So I've been doing a little bit of work on that. And that's been quite interesting. So I use a tool called edge impulse. And a lot of it goes on to either the Raspberry Pi or the ESP 32.
Peter: Yeah. Well, what kind of signals are those like we're talking about visual, audible, or say, like, temperature, humidity, things of that sort?
Philip: Well, as an example, I can get the game going, scissors, paper and rock going, either with a camera, or even simpler, just using a photo electric cell. And with a photo electric cell, it's able to look at the patterns from the fingers, and actually make some interpretation of what the gesture might be for the game. So you can actually simplify the sensors, but you look at patterns, time sequences, or patterns to make some inference.
Peter: So I guess, you're using the edge AI, which is able to make those inferences on the device itself without having to communicate with a large AI model like chat GPT, which lives somewhere on the cloud. So you can train the inference model or the inference engine on the device itself, right? So it stays local. And I guess it's fast. Is that what the objective is there?
Philip: Yeah, the objective is to make it as reliable as possible. In certain circumstances, you might not have the internet available, or maybe down. That doesn't mean to say that your application has to totally shut down. You can still operate, particularly in critical situations where there might be safety involved. And so it's really a mixture. It's been able to get the best out of the internet and cloud services, but at the same time, be able to make it reliable.
Peter: Right. So it's a hybrid model. What is the underlying technology behind edge AI? Is it like neural networks, expert systems? And do you teach those fundamentals to the students?
Philip: Not with Chatsworth High School, we actually did. We went through that. And the teachers there were also teaching years 11 and 12, and wanted to use some of the concepts that we were exploring for the older students. I thought that was interesting. But yeah, we're using neural networks. And we're teaching the machines patterns. So there's a pattern there that we go through in identifying the signals that we're interested in and associating those in a teaching model. So it's actually quite simple. It doesn't involve higher level databases. We have very, very simple for the edge AI, it's actually very simple. And for a thing where I start using a camera, then I need a much larger data set with bigger and quite often for rubbish, I scout around the internet, and I use other data sets and build on top of what other people have also done.
Peter: It's interesting. As I see it, based on your description, you're basically teaching students, humans, how to teach a machine to learn through, in this case, you mentioned rubbish by looking on the internet for photography images of rubbish that you can use as training material for the edge AI. So you're teaching humans how to teach machines that the machines can then make decisions that can help humans out in this case with recycling of rubbish. Is that right?
Philip: Well, that's the problem was the school wasn't managing its rubbish program very well. And the concept that I had here was that if the students were getting feedback, it could improve their behavior. If they were putting plastic in a compost bin, for example, the computer could identify and tell students that that's incorrect. So it was a cycle.
Peter: So are you actually building some kind of rubbish bin that can see what kind of rubbish is being put in it and provide feedback to the student or to whoever puts rubbish in as to incorrect bin?
Philip: The models that we went through are still desktop models. They were intended to go out into the playground later on. But that would have to go through a couple of extra steps. And one of those would be to improve the communications. So we might even use security cameras instead of the PC cameras, which are in the classroom. And I do that at home, I've got my security cameras all wired up into an artificial intelligence. So it means that I've got the cameras wherever I want them. And also for communications, the cameras are very close. So there's no problem there with the communications. But outside, if you've got a remote camera, you might have to look at other forms of communicating. And one of those was we're exploring the use of Lora Wang for a big school that had quite a distributed area. So that was one concept.
Peter: So for long range communications, but if I understand right, you're talking about communicating the inference that the Edge AI has made about, for example, a particular type of garbage being put in a bin, communicate that information of correct or incorrect, I guess, allocation of garbage into bin to the student, right?
Philip: That's right. Yeah, the idea would be that anywhere in the school, which has got quite a wide area for playgrounds and sports fields, that we could put a camera anywhere, and the camera could make decisions. And only the decisions, not the image would be fed back to a login center.
Peter: Right, so then you could collect statistics on, for example, how many correct or incorrect deposits of garbage in bins have been made. And then that information can be used by students and staff to infer on the, I guess, correct use of recycling bins, right?
Philip: That's right. It would give us an overall picture, considering the fact that we had quite a wide area to cover. It's an interesting application. Yeah, sorry. I'm just thinking. Our project was only still desktop based. And at the desktop, it relied on the feedback from either the Microsoft or the Google model. Now, the Google one, I actually took it a little bit further, so that students could be interested in their environment. And the way I did that was to have a database of bird calls. And so the students were able to identify the species of birds by the sounds that the birds were making. The Google one not only allows you to use images, but it also allows you to use sounds. And I've got a complete model now. Someone else has done this on a Raspberry Pi. And we've now got a program called BirdNet working that can identify all the birds in our local area.
Peter: So we've got a microphone connected to our Raspberry Pi, which will listen out for birds and then just, I guess, on the screen, it will tell you what bird it is.
Philip: Well, we've got about how many cameras in our house, perhaps about five or six cameras, security cameras. And the audio of all of those goes into the BirdNet data logger. So we've got the whole property surrounded. And it's quite interesting because we've made about 10,000 sightings over two months and identified, I thought there was only about one or two species of birds, but there was 26 species of birds identified using this model.
Peter: In your neighbourhood?
Philip: Yeah, in the neighbourhood. Now, the purpose behind this was to connect students with nature and with the environment so that there'd be a closer appreciation and they'd be able to see what's happening and know a little bit more what's happening outside. I thought that was quite an interesting extension. It's a separate project, but it's also part of the model that I'm interested in.
Peter: That's very interesting. So I guess there you are covering quite a wide spectrum of what applications AI can have in daily life. You've got video, you've got the audio, you could obviously put in other sources of signals, various sensors, it could be atmospheric sensors, humidity, temperature, various gases. So you could use all that to, I guess, automate the world around us and extract information that can be used to make decisions, in this case, on environmental impacts of our activities.
Philip: Yeah, it's not only environmental. I've also applied it in our STEM club for music. And that was quite interesting because the kids there, I try to make connections of what they're interested in. And some of them are interested in football. So we said, "Oh, well, how about we use AI to come up with the theme song for your local football club? And we'll also come up with a new logo for your football club, only using the colours of your football club, without telling what those colours are." And what's interesting, it was able to, the machine that we used was ChatGDP. And also, I used Barb too. But some of the kids had never really experienced what AI is like. They might have heard about it, their parents talking about it. But here, we actually made a connection of what they could be interested in. And they were really interested in what was happening.
Peter: So you link it to something that is very interesting to them, like football and the football team. And then you apply it to artificial intelligence, they're immediately interested in the outcome. Because of these two topics now play together. It's not just an abstract application of AI in some topic that they're not interested in. So it's very, very clever. You need to know who you're talking to, right? In education, it's like a support in every other part of life, you need to know your audience.
Philip: Exactly. The other thing was that I'm covering quite a wide range. Most of the coding that we do, uses Blocky or MakeCode, which is quite good for younger kids. But then I talk about Python, and they can see how the Blocky is translated. But then I say, well, if you tell chat GDP, what you want, it could, and particularly if you tell it that it's for a micro bit computer, it can come up with the code. And we did that with music, we said, create a program for a micro bit computer that can play music. And again, they were quite interested in that, because they saw two different ways of doing it. One way they did it on MakeCode. And this other way, all they did was to concentrate on the requirements, or the objective. And they could express that into chat GDP. And it came up with some interesting answers.
Peter: It's a good start, isn't it? Like you can get you can use prompts in chat GPT to get you started with something that you want to build. Like create the first draft of the first prototype, and then you can improve from there. But I guess if you look at it as an assistant, in education as well as well as in other parts of creative life, that that can be a way for students to get started learning a topic using chat GPT as an assistant.
Philip: Yeah. And being having the role of an assistant is quite important there. Because you don't want it to do the work for you. You really have to have a conversation and correct what you think might be a problem with the code that it gives you back. And I think what's interesting, I took this to another level just yesterday. My son, he's quite an expert. He works for the CSI road. And we collaborate a little bit. And we did this yesterday. And he told chat GDP that the code that he for controlling cameras on our security system was inadequate. And after about nine iterations, he eventually got chat GDP to come up with code, which was workable.
Peter: Yeah, yeah. I've got similar experience with that as well. I've used to GPT to help me write Ruby code, quite a lot of it in the last couple of months. And yeah, it often starts with a prototype that doesn't quite do what I wanted, sometimes got bugs. But through a few iterations, just like you do with your own code, it eventually gets there. And the really interesting thing for me from a programming perspective, is that iterating with chat GPT help is much faster than iterating using the usual Googling around method. It's just it's just much faster. It won't write the code for you, but it will help you get there much faster than without it.
Philip: And so as an example, with students, you really have to go through that iteration to show them that the first results that you get might not be satisfactory, and that they shouldn't submit that in their own assignments, for example. But the role might be that they could use chat GDP as a someone to check their their final code, and then make a statement about the feedback that they get from that.
Peter: Exactly. It also you can also ask chat GPT to analyze code, explain how it works, explain particular lines of code, or explain the whole thing or even you can get it to rewrite a piece of code that you've already written, but make it better make it more efficient. We can we can parameterize it and say make it better in this way, for example, make it faster, improve the variable names can even do that. So find that also amazing capability. Also from our education point of view. Another thing that I've done is code that I do not understand. Because for example, I got it from someone's GitHub repository, I put it in chat GPT and ask it to explain it to me explain what it does for a student that is also amazing.
Philip: Yeah, it's got a long way to go. In the STEM club environment. And also, there's been a recent